Collaborative AI Agents in Software Testing: The Developer Path to Code Quality
This is my second article in the journey of AI for testing following From AI Assistants to Collaborative Agents: The Future of Software Testing.
Are developers becoming obsolete? For decades, developers were some of the most sought-after professionals. Organizations depended on manually-written code to deliver features to customers and value to their respective businesses. Consequently, the power to make or break a company’s business success was literally in their hands.
But today, AI tools are becoming increasingly capable of writing, debugging and testing code. Now, many technological prophets claim that AI will replace developers, and developers are beginning to fear for their job security.
I don’t believe we’re in a such crisis, but we’re surely at a juncture. AI cannot completely replace developers in the near future, since the generated code is ridden with errors and vulnerabilities. Humans will always be needed throughout the process either as validators, operators, or chime in to handle cases where AI got it wrong.
However, with human guidance, AI can support and power software development, helping developers write faster and higher quality code and tests. In fact, I believe it will make them better professionals, with higher job market attractiveness.
In this article, we’ll explore the concept of joint AI and human developer collaboration, called “collaborative AI agents”. We’ll share when and how to use collaborative AI agents for software testing, provide prompting ideas and explain how to get started.
Quick Reminder: What Are Collaborative AI Agents in Software Testing?
Collaborative AI Agents are specialized, task-oriented AI systems designed to work alongside humans to accomplish tasks from end-to-end. On our technological path to fully agentic systems, collaborative AI agents partner with humans who guide them and provide clarity and examples, so agents can deliver outcomes of the utmost quality.
In the future, when infrastructure support allows, collaborative AI agents will be replaced by fully-capable agents. Then, we’ll see agents collaborating with each other. (And even then, human developers will still be in the loop).
In software testing, collaborative AI agents can write code, generate tests and suggest fixes. Humans lead by refining test generation with clear prompts and focus on areas of the code that matters more.
When to Use Collaborative Agents in Software Testing
Since automated and manual testing is slow, error-prone and siloed, AI has entered as a solution to help developers enhance test quality. Collaborative AI agents can understand code structure, logic and business rules, meaning they can support any type of testing developers conduct.
Think of these agents as highly capable teammates — they excel at their job but still need direction. Unlike assistants that wait for step-by-step instructions, collaborative AI agents will do a good job with whatever task they're given. However, they may not complete it fully if they're missing some key context or simply can't read the mind of the human operator.
Collaborative AI agents can be used in any part of the SDLC where testing takes place. This includes unit testing during early-stage development, integration, system, regression and performance testing before deployment and regression testing when new code is introduced.
For each type of test, these Agents can be used for generating initial tests, testing edge cases or human-inputted priorities, generating mocks and synthetic test data, expanding the test suite, covering functionality, performance and security needs, for PR shipping or for entire repositories.
Soon, collaborative AI agents can also help analyze the root cause of bugs. This is based on fast and sophisticated analysis of code, data, and logs from existing and previous incidents as well as public GitHub repos.
Finally, collaborative AI agents can be incorporated as part of CI/CD processes. This will ensure continuous feedback and that AI is always part of the testing process as the code evolves.
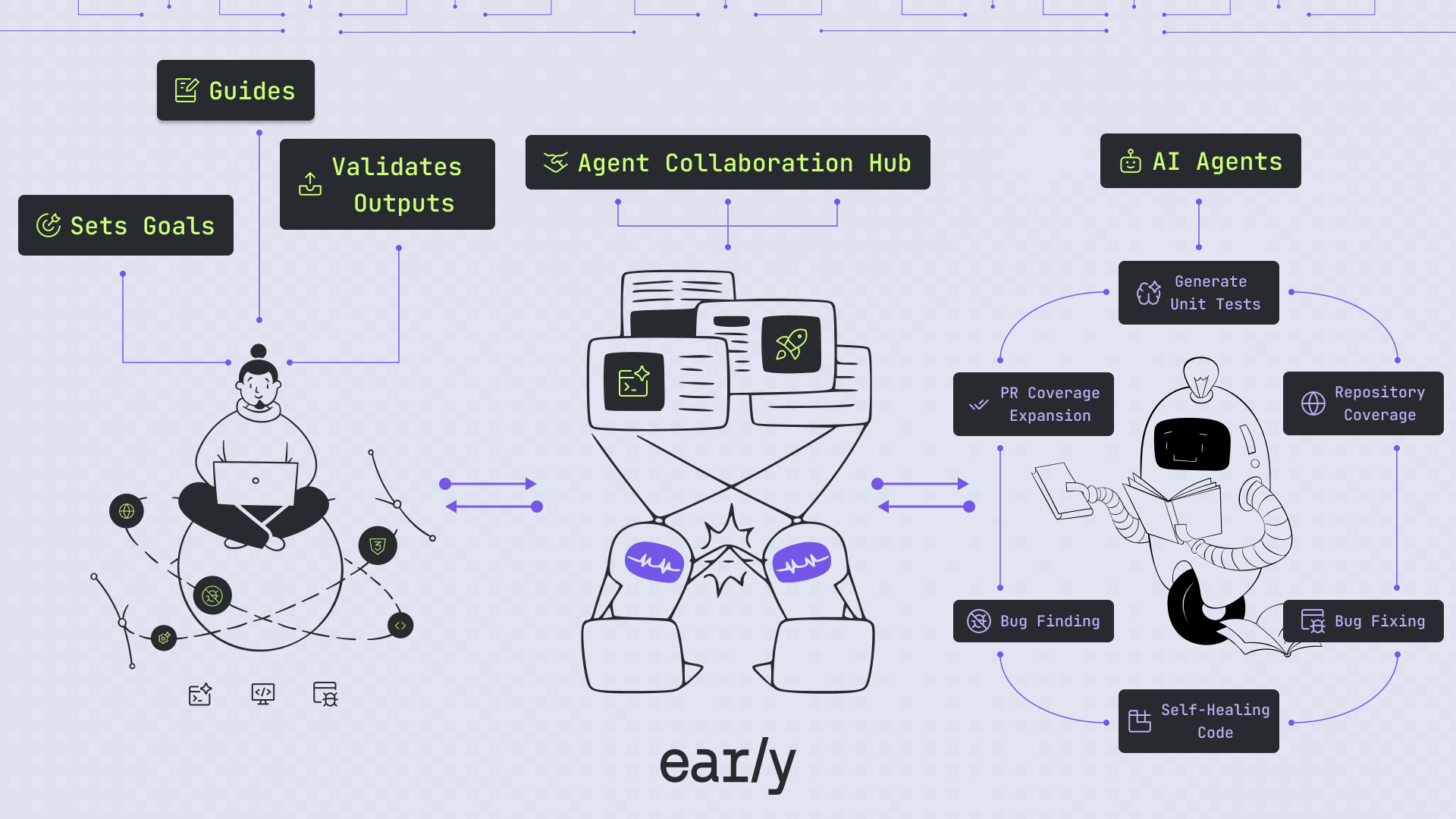
Pitfalls to Avoid
Sounds wonderful, right? It is. But just in case, here’s what not to do when working with collaborative AI agents in your testing:
- Using AI Assistants vs. Agents - Despite the industry-wide confusion, collaborative AI agents are not the same as AI assistants. AI assistants are reactive tools that handle tasks like code autocompletion, answering questions, and generating snippets or large chunks of code. However, they require continuous oversight and guidance just to produce code that functions as expected or simply working code. Their outputs can include duplicated code, which creates long-term maintenance and quality challenges. They also have a tendency to hallucinate or deliver superficial solutions that lead to high error rates. In contrast, AI agents act, adapt, and follow through like true teammates. They possess reasoning capabilities, making them significantly more autonomous. As a result, they often deliver higher-quality outcomes on the first attempt.
- Lack of human-in-the-loop - AI agents are autonomous, not collaborative. Leaving them without guidance and clarifications can result in suboptimal results. This can lead to wasted time or misaligned testing priorities. Remember, they don’t replace humans, they augment them.
- Poor guidelines - Weak or vague directions (e.g. followup prompts) will result in bad outputs. Take the time to invest in your prompts, and refine them as needed,
Here are some sample prompts you can use to start with:
- Add more edge cases, especially for invalid inputs.
- Refactor the tests to use beforeEach for setup.
- Improve mock usage to avoid unnecessary function calls.
- Add test cases for when the API call fails.
- Use this example data to generate more tests alike for this input attribute
- Expand coverage to include timeout and network error scenarios.
- Verify that error messages are user-friendly and actionable.
- Include tests for different user permission levels.
- Write tests that simulate slow or unstable network conditions.
- Use parameterized tests to check multiple input variations.
- Ensure error responses don’t leak sensitive system information.
Best Practices for Collaborative AI Agent Success
Based on my experience in using software testing with AI generated code, here are some tips I believe can help you get better results from your AI agents:
- Be specific when asking for improvements. What needs to be better, and why?
- Try iterative changes: start with one improvement, then add more. This will allow you to test what’s working and what isn’t for future improvements.
- Provide structured context that the system might not have like system architecture, recent bugs, user story, or business rules, if they are not apparent in the code or the agent hasn’t used them sufficiently.
- For repetitive section of the prompt use rules, or guideline across all tests (user level, repo level, company level)
How to Get Started
Here’s a simple path to onboarding collaborative AI agents:
- Pick a use case: Start small, like writing unit tests for one of your code methods, don’t pick something too easy, AI power comes in the more complex scenarios.
- Explore platforms that offer AI agents tailored to specific tasks or units of work (such as EarlyAI for unit testing). Remember, agents are designed to excel at a particular function — the more general-purpose the tool, the more mediocre the results are likely to be.
- Run pilots: Run the first tests and compare agent performance to existing code generation tools or human baselines.
- Refine: Update the prompts to enhance tests on areas where the agent lacked information.
- Integrate into workflows: Plug your agent into CI/CD, MCP workflow, or external sources of data the agent can use for better results.
- Measure outcomes: Track the quality of the tests, issues caught, quality of bugs found, test coverage improvements, and time saved for delivering your pull requests.
Final Thoughts
The future of development isn't about replacing developers. It's about augmenting them — helping them build better, faster, smarter.
Collaborative AI Agents are the next step forward. And if you embrace them early, you’ll move faster than ever — and stay ahead of the curve.